Listen with Care: Exploring the Ethics of Speech Emotion Recognition Technology
- Auxane Boch
- Nov 19, 2023
- 3 min read
Welcome AI Ethics Enthusiasts!
Artificial Intelligence can analyse the audible aspects of speech to detect emotions, which is awesome! In this edition, we'll dive into the captivating world of Speech Emotion Recognition (SER) and explore its exciting applications in different fields.
What to Know?
SER is about using AI techniques to predict human emotions from audio signals. This technology has practical uses in psychology, medicine, education, and entertainment, among others. To accurately identify emotions, we need to extract relevant features from audio signals, which is a crucial step in the SER process.
Regarding recognising emotions in speech, there are two main research areas. The first focuses on creating artificial emotional sounds, while the second aims to recognise the emotional states of individuals. Machines need to learn about human emotions from speech, and that's where classifiers come in. These classifiers analyse speech-based data to identify emotional cues and extract the necessary features.
A typical system for recognising emotions in speech consists of several interconnected processes and elements. The first step is gathering data, which can involve recording actors expressing different emotions while reading the same text. Researchers also rely on existing databases created by others. Sometimes, a hybrid approach that combines both sources is used to build more comprehensive models.
Next, we move on to feature extraction from the collected or acquired data. Acoustic features include intonation, intensity, linear prediction, and formants. Linguistic features, on the other hand, encompass phonemes, words, paralinguistics (like laughter or sighs), and disfluencies (such as pauses). Non-linguistic factors like the speaker's age, gender, and emotional state also play a role.
Specialised software can effectively analyse voice features and generate waveforms, spectrograms, pitch tracks, apply filters, perform processing and segmentation, and more. This type of software provides valuable insights into the speech signal.
Once the features are extracted, we enter the realm of machine learning. Statistical relationships between specific features and emotional states are analysed and identified. This part is often called the "classifier," as it learns to classify emotions based on the extracted features.
Let's Discuss Ethics
As we explore the potential of AI in SER, it's essential to consider the ethical challenges that come with it.
Accuracy is a crucial ethical concern. Ensuring that emotion recognition algorithms are accurate is essential. Misidentifying or misinterpreting emotions can lead to misunderstandings or inappropriate responses. For example, misclassifying someone's speech as anger when they're actually expressing enthusiasm could result in unnecessary conflict or strained relationships.
Transparency is also vital. It's essential to be transparent about emotion recognition technology and inform individuals when their speech is being analysed. Clear guidelines and consent mechanisms should be in place to protect privacy and autonomy. For instance, employers using emotion recognition technology in the workplace should communicate its purpose, scope, and implications to employees to avoid feelings of surveillance or invasion of privacy.
Data privacy is another significant ethical consideration. Emotion recognition systems rely on collecting and analysing audio data, so safeguarding this data is critical. It's essential to prevent unauthorised access or misuse. For example, voice assistants with emotion recognition technology should have robust security measures to ensure that personal conversations aren't recorded or stored without explicit consent.
As much as there are challenges, ethical opportunities also arise from using SER.
Use Cases Matter
In healthcare, emotion recognition can be instrumental. It can help identify emotional states in patients experiencing pain, anxiety, or depression, enabling healthcare professionals to provide better support and tailored interventions. For example, during telemedicine consultations, emotion recognition technology can help doctors assess a patient's emotional well-being, leading to appropriate care and referrals if necessary.
In education, emotion recognition can assist in understanding individual students' emotional states. This allows educators to provide targeted support and adapt teaching approaches accordingly when the student is exchanging with an AI-powered robot. For instance, if emotion recognition technology can identify signs of confusion or frustration in a student's speech, the teacher can use additional explanations or alternative learning strategies to enhance comprehension.
In conclusion, AI's ability to recognise emotions in speech opens up a world of possibilities across various domains. By analysing audible speech patterns, inflexion, emphasis, and talking speed, we can gain deeper insights into human emotions. As we move forward, it's crucial to address ethical concerns and seize the opportunities to apply this technology responsibly to benefit individuals and society. So, let's embrace the potential of emotion recognition technology and make a positive and responsible impact!
If you have any questions or thoughts, feel free to reach out!
Until next time,
- Auxane Boch
References and Interesting Reads
N. K. R. Almarzooqi, "Speech emotion recognition framework and applications in emergency call centers," The 3rd International Conference on Distributed Sensing and Intelligent Systems (ICDSIS 2022), Hybrid Conference, Sharjah, United Arab Emirates, 2022, pp. 321-329, doi: 10.1049/icp.2022.2482.
Alluhaidan, A. S., Saidani, O., Jahangir, R., Nauman, M. A., & Neffati, O. S. (2023). Speech Emotion Recognition through Hybrid Features and Convolutional Neural Network. Applied Sciences, 13(8), 4750. https://doi.org/10.3390/app13084750
Saif M. Mohammad; Ethics Sheet for Automatic Emotion Recognition and Sentiment Analysis. Computational Linguistics 2022; 48 (2): 239–278. doi: https://doi.org/10.1162/coli_a_00433
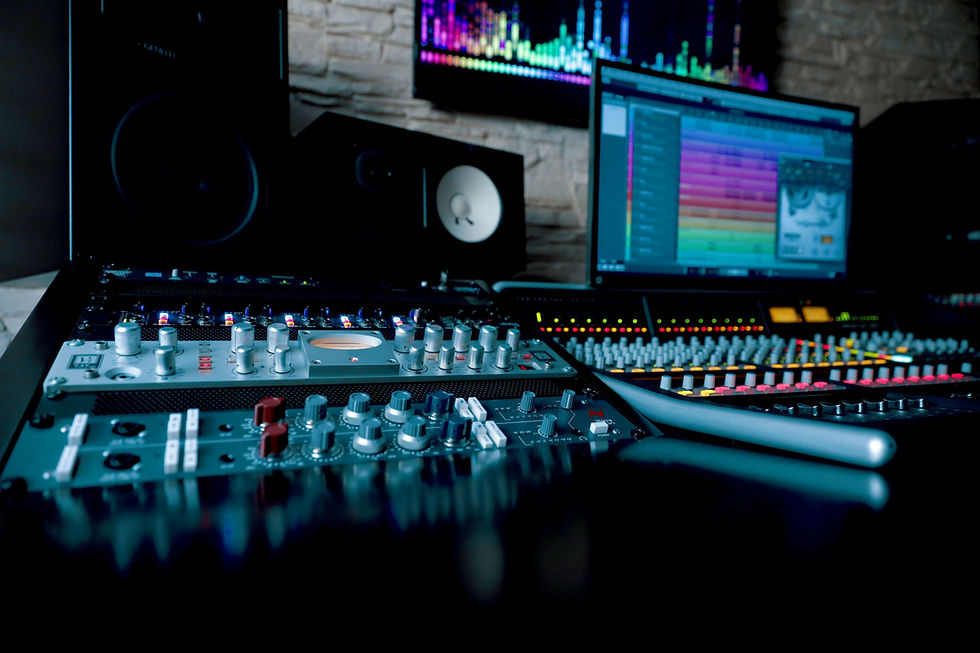
Comments